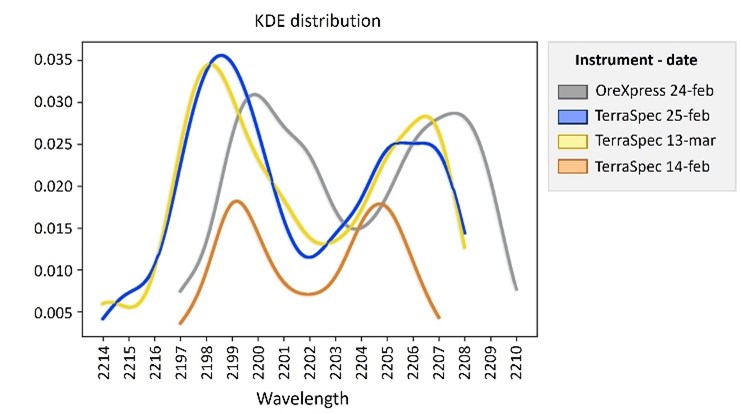
Published
Evaluation of normalization methods applied to Short-Wavelength Infrared (SWIR) spectroscopy mineral databases from multiple instruments and for vectoring analysis exploration
Evaluación de métodos de normalización aplicados a bases de datos minerales de Espectrografía de Infrarrojo Cercano (SWIR) provenientes de múltiples instrumentos y para análisis de vectores de exploración
DOI:
https://doi.org/10.15446/rbct.n56.113445Keywords:
Reflectance spectroscopy, SWIR, White mica, database normalization (en)Espectroscopía de reflectancia, SWIR, Mica blanca, normalización de bases de datos (es)
Downloads
Over the past decade, short-wave infrared (SWIR) spectroscopy has made significant advances in detecting geochemical variations in minerals like white mica, alunite, and chlorite for exploring hydrothermal ore deposits. These variations provide valuable clues, indicating changes in temperature, pH, and fluid oxidation state towards the mineralized center. However, small calibration differences among devices challenge data integration. This study evaluates the 2200 nm Al-OH absorption feature in four white mica SWIR spectroscopy databases collected by TerraSpec™ and OreXpress™ from samples at the Grasshopper porphyry prospect. It evaluates three normalization methodologies: rescaling, mean normalization, and Z-score, yielding p-values for successful data merging of up to 0.75. Findings suggest effective normalization methods across devices, reducing biases from uncalibrated spectrometers. This research offers a methodology to correct SWIR database biases, facilitating accurate data integration across instruments for vectoring analysis.
Durante la última década, la espectroscopía de infrarrojo de onda corta (SWIR) ha experimentado avances significativos en la detección de variaciones geoquímicas en minerales como la mica blanca, la alunita y la clorita para explorar depósitos de minerales hidrotermales. Estas variaciones proporcionan pistas valiosas, indicando cambios en la temperatura, el pH y el estado de oxidación del fluido hacia el centro mineralizado. Sin embargo, las pequeñas diferencias de calibración entre dispositivos representan un desafío para la integración de datos. Este estudio evalúa la característica de absorción del Al-OH a 2200 nm en cuatro bases de datos de espectroscopía SWIR de mica blanca recopiladas por TerraSpec™ y OreXpress™ a partir de muestras en el prospecto de pórfido Grasshopper. Se analizan tres metodologías de normalización: reescalado, normalización de la media y variable centrada reducida, obteniendo valores de p para la fusión exitosa de datos de hasta 0.75. Los hallazgos sugieren métodos de normalización efectivos entre dispositivos, reduciendo sesgos de espectrómetros no calibrados. Esta investigación ofrece una metodología para corregir sesgos de la base de datos SWIR, facilitando la integración precisa de datos entre instrumentos para análisis de vectores.
References
[1] Cohen, J.F., Compositional variations in hydrothermal white mica and chlorite from wall-rock alteration at the Ann-Mason porphyry copper deposit, Nevada, 2011.
[2] Halley, S., Dilles, J.H., and Tosdal, R.M., Footprints: hydrothermal alteration and geochemical dispersion around porphyry copper deposits. SEG Discovery, (100), pp. 1-17, 2015. DOI: https://doi.org/10.5382/SEGnews.2015-100.fea
[3] Clark, R.N., King, T.V., Klejwa, M., Swayze, G.A., and Vergo, N., High spectral resolution reflectance spectroscopy of minerals. Journal of Geophysical Research: Solid Earth, 95(B8), pp. 12653-12680, 1990. DOI: https://doi.org/10.1029/JB095iB08p12653
[4] Pontual, S., Merry, N., and Gamson, P., Spectral interpretation field manual, G-MEX edition 3. Spectral Analysis guides for mineral exploration. 1997.
[5] Thompson, A.J., Hauff, P.L., and Robitaille, A.J., Alteration mapping in exploration: application of short-wave infrared (SWIR) spectroscopy. SEG Discovery, (39), pp. 1-27, 1999. DOI: https://doi.org/10.5382/SEGnews.1999-39.fea
[6] Chang, Z., Hedenquist, J.W., White, N.C., Cooke, D.R., Roach, M., Deyell, C.L., ... & Cuison, A.L., Exploration tools for linked porphyry and epithermal deposits: example from the Mankayan intrusion-centered Cu-Au district, Luzon, Philippines. Economic Geology, 106(8), pp. 1365-1398, 2011. DOI: https://doi.org/10.2113/econgeo.106.8.1365
[7] Xiao, B., Chen, H., Hollings, P., Wang, Y., Yang, J., and Wang, F., Element transport and enrichment during propylitic alteration in Paleozoic porphyry Cu mineralization systems: Insights from chlorite chemistry. Ore Geology Reviews, 102, pp. 437-448, 2018. DOI: https://doi.org/10.1016/j.oregeorev.2018.09.020
[8] Uribe-Mogollon, C., and Maher, K., White mica geochemistry of the Copper Cliff porphyry Cu deposit: Insights from a vectoring tool applied to exploration. Economic Geology, 113(6), pp. 1269-1295, 2018. DOI: https://doi.org/10.5382/econgeo.2018.4591
[9] Neal, L.C., Wilkinson, J.J., Mason, P.J., and Chang, Z., Spectral characteristics of propylitic alteration minerals as a vectoring tool for porphyry copper deposits. Journal of Geochemical Exploration, 184, pp. 179-198, 2018. DOI: https://doi.org/10.1016/j.gexplo.2017.10.019
[10] Chang, Z., and Yang, Z., Evaluation of inter-instrument variations among short wavelength infrared (SWIR) devices. Economic Geology, 107(7), pp. 1479-1488. 2012. DOI: https://doi.org/10.2113/econgeo.107.7.1479
[11] Uribe-Mogollon, C., and Maher, K., White mica geochemistry: Discriminating between barren and mineralized porphyry systems. Economic Geology, 115(2), pp. 325-354, 2020. DOI: https://doi.org/10.5382/econgeo.4706
[12] Silverman, B.W., Density estimation for statistics and data analysis. Routledge, New York, 2018, 176 P. DOI: https://doi.org/10.1201/9781315140919
[13] Danese, M., Lazzari, M., Murgante, B., Integrated geological, geomorphological and geostatistical analysis to study macroseismic effects of 1980 Irpinian Earthquake in urban areas (Southern Italy). In: Gervasi, O., Taniar, D., Murgante, B., Laganà, A., Mun, Y., Gavrilova, M.L., (eds), Computational Science and Its Applications – ICCSA 2009. ICCSA 2009. Lecture Notes in Computer Science, vol 5592. Springer, Berlin, Heidelberg. 2009. DOI: https://doi.org/10.1007/978-3-642-02454-2_4
[14] Barnett, R.M., and Deutsch, C.V., Multivariate imputation of unequally sampled geological variables. Mathematical Geosciences, 47(7), pp. 791-817, 2015. DOI: https://doi.org/10.1007/s11004-014-9580-8
[15] Tian, X., Kong, Y., Gong, Y., Huang, Y., Wang, S., and Du, G., Dynamic geothermal resource assessment: integrating reservoir simulation and Gaussian Kernel density estimation under geological uncertainties. Geothermics, 120(103017), art. 103017, 2024. DOI: https://doi.org/10.1016/j.geothermics.2024.103017
How to Cite
APA
ACM
ACS
ABNT
Chicago
Harvard
IEEE
MLA
Turabian
Vancouver
Download Citation
License
Copyright (c) 2024 Boletín de Ciencias de la Tierra

This work is licensed under a Creative Commons Attribution-NonCommercial-NoDerivatives 4.0 International License.
The author of a paper accepted for publication in any of the journals published by the School of Mines will yield all the property to the National University of Colombia rights free of charge, within which include article: the right to edit, publish, reproduce and distribute both print and digital media, as well as to include article in international indexes and / or databases, likewise, empowering the publisher to use images, tables and / or graphic material presented in Article for designing covers or posters of the magazine. By assuming economic rights of the article, may be reproduced partially or totally in any printed or digital media without express permission of the same.