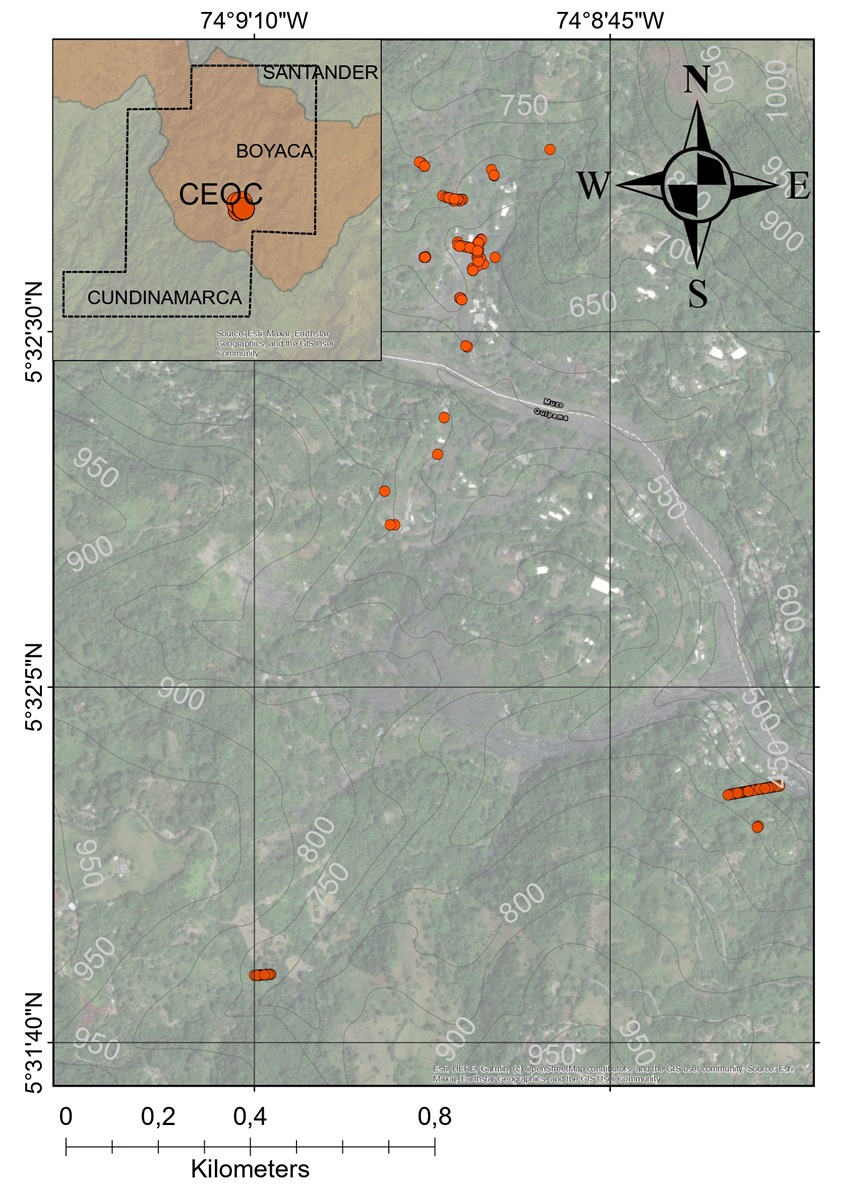
Publicado
Estimation of emerald mineralization probability using machine learning algorithms
Estimación de la probabilidad de mineralización de esmeraldas usando algoritmos de aprendizaje automático
DOI:
https://doi.org/10.15446/dyna.v92n235.112504Palabras clave:
gems, calibration, drillhole, mineral target (en)gemas, calibración, perforación, objetivo minero (es)
Descargas
This research proposes a machine learning (ML) model that estimates the probability of emerald mineralization in rocks of the Western Emerald Belt (CEOC). Element concentrations, lithologies and coordinates were used as input variables and productivity as the target variable (176 samples). The variables were transformed to be integrated into the model. (1) Variable selection was performed using the Boruta method and backward elimination. (2) A logistic regression, a neural network, and a support vector machine were trained. (3) Calibration was achieved with the Platt method. (4) Calibration assessment was conducted by using the Brier score and calibration curves. The model selected was a calibrated support vector machine (C = 0.19 and λ = 0.1) that included 17 geochemical variables and the coordinates. The results were presented in a 3D plot. Assigning a probability value to each sample allows the mining targets to be ranked.
La investigación propone un modelo de aprendizaje automático para estimar la probabilidad de mineralización de esmeraldas en el Cinturón Esmeraldífero Occidental (CEOC). Se emplearon concentraciones elementales, litología y coordenadas como variables de entrada y la productividad como variable objetivo (176 muestras). Las variables fueron transformadas para ser integradas al modelo. (1) Se recurrió a los métodos Boruta y backward elimination para seleccionar las variables. (2) Una regresión logística (LR), una red neuronal de retropropagación (BPNN) y una máquina de vectores de soporte (SVM) fueron entrenadas. (3) Se usó la calibración de Platt y (4) se evaluó su desempeño usando la puntuación Brier y curvas de calibración. El modelo elegido fue una máquina de vectores de soporte calibrada (C = 0.19 y λ = 0.1) que incluyó 17 variables geoquímicas y las coordenadas. Asignar valores de probabilidad permitió jerarquizar los objetivos mineros.
Referencias
[1] Cartier, L.E., Gemstones and sustainable development: perspectives and trends in mining, processing and trade of precious stones. Extr Ind Soc. 6(4), pp. 1013-1016, 2019. DOI: https://doi.org/10.1016/j.exis.2019.09.005
[2] Agencia Nacional Minera - Unidad de planeación minero-energética UPME. Producción histórica de esmeraldas en Colombia. [online]. 2021.
[3] Dominy, S.C., Noppé, M.A., and Annels, A.E., Errors and uncertainty in mineral resource and ore reserve estimation: the importance of getting it right. [online]. 2004. Available at: https://bit.ly/3os0e4M. DOI: https://doi.org/10.2113/11.1-4.77
[4] Quintín, J., Estudios de la estimación y simulación geoestadística para la caracterización de parámetros geólogo-industriales en el yacimiento laterítico Punta Gorda. Minería y Geología. 21, 2005.
[5] Niño, G., and Sheng-Rong, S., Geological and geochemical analyses for emerald exploration in the Muzo Formation along the Western Emerald Belt, Colombia. National Taiwan University, 2017.
[6] Melo, R.T., Notas sobre el contexto tectonoestratigráfico de formación de las esmeraldas colombianas. Boletín Geológico. 45(45), pp. 37-48, 2019. DOI: https://doi.org/10.32685/0120-1425/boletingeo.45.2019.486
[7] Moreno, G., Terraza, R., Montoya, D., Geología del cinturón esmeraldífero oriental. Boletín de Geología. [online]. 31, pp. 51-67, 2009. Available at: https://www.scielo.org.co/scielo.php?script=sci_arttext&pid=S0120-02832009000200004&nrm=iso
[8] Pignatelli, I., Guiliani, G., Ohnenstetter, D., et al., Colombian Trapiche Emeralds: recent advances in understanding their formation. Gems & Gemology. 51, pp. 222-259, 2015. DOI: https://doi.org/10.5741/gems.51.3.222
[9] Groat, L.A., Giuliani, G., Marshall, D.D., Turner, D., Emerald deposits and occurrences: a review. Ore Geol Rev. 34(1-2), pp. 87-112, 2008. DOI: https://doi.org/10.1016/j.oregeorev.2007.09.003
[10] Mendoza, J., Anotaciones geoquímicas para exploración de esmeraldas en la región Muzo-Coscuez con base en la relación Na/K y elementos traza. Geología Colombiana. 21, pp. 89-98, 1996.
[11] Ottaway, T.L., The geochemistry of the Muzo emerald deposit, Colombia. University of Toronto, Toronto, Canada, 1991, 216 P.
[12] Ochoa, L.H., Evaluación magnetometría, radiométrica y geoeléctrica de depósitos esmeraldíferos. Geofísica Colombiana. 7, pp. 13-18, 2003.
[13] Dumakor-Dupey, N.K., and Arya, S., Machine learning—a review of applications in mineral resource estimation. Energies (Basel). 14(14), art. 4079, 2021. DOI: https://doi.org/103390/en14144079
[14] Zhang, N., Zhou, K.,and Li, D., Back-propagation neural network and support vector machines for gold mineral prospectivity mapping in the Hatu region, Xinjiang, China. Earth Sci Inform. 11(4), pp. 553-566, 2018. DOI: https://doi.org/10.1007/s12145-018-0346-6
[15] Mahvash-Mohammadi, N., and Hezarkhani, A., Application of support vector machine for the separation of mineralised zones in the Takht-e-Gonbad porphyry deposit, SE Iran. Journal of African Earth Sciences. 143, pp. 301-308, 2018. DOI: https://doi.org/10.1016/j.jafrearsci.2018.02.005
[16] Taboada, J., Matías, J.M., Ordóñez, C., and García, P.J., Creating a quality map of a slate deposit using support vector machines. J Comput Appl Math. 204(1), pp. 84-94, 2007. DOI: https://doi.org/10.1016/j.cam.2006.04.030
[17] Bonilla, G., Castaño, A., Nieto, M.A., and Parra, S., Guía de buenas prácticas de la esmeralda colombiana. Published online 2020.
[18] Platt, J.C., Probabilistic outputs for support vector machines and comparisons to regularized likelihood methods. Advances in Large Margin Classifiers. 10(3), pp. 61-74, 1999.
[19] Kursa, M., Jankowski, A., and Rudnicki, W., Boruta - A system for feature selection. Fundam Inform. 101, pp. 271-285, 2010. DOI: https://doi.org/10.3233/FI-2010-288
[20] Nguyen, H.B., Xue, B., Liu, I., and Zhang, M., Filter based backward elimination in wrapper based PSO for feature selection in classification. In: 2014 IEEE Congress on Evolutionary Computation (CEC). 2014, pp. 3111-3118. DOI: https://doi.org/10.1109/CEC.2014.6900657
[21] Kaplan, D., Bayesian statistics for the social sciences. The Guilford Press. 2014, 318 P.
[22] Guo, C., Pleiss, G., Sun, Y., and Weinberger, K.Q., On Calibration of modern neural Networks. Proceedings of the 34th International Conference on Machine Learning. Vol. 70, 2017. DOI: https://doi.org/10.48550/ARXIV.1706.04599
[23] Niculescu-Mizil, A., and Caruana, R., Obtaining Calibrated probabilities from Boosting. In: UAI. Vol 5, 2005, pp. 413-420.
[24] Brier, G.W., Weather review verification of forecasts expressed in terms of probability. Monthy Weather Review. [online]. 78(1), pp. 1-3, 1950. [Accessed November 13th, 2023]. Available at: https://journals.ametsoc.org/view/journals/mwre/78/1/1520-0493_1950_078_0001_vofeit_2_0_co_2.xml. DOI: https://doi.org/10.1175/1520-0493(1950)078<0001:VOFEIT>2.0.CO;2
[25] Roulston, M.S., Performance targets and the Brier score. Meteorological Applications. 14(2), pp. 185-194, 2007. DOI: https://doi.org/10.1002/met.21
[26] Niu, L., A review of the application of logistic regression in educational research: common issues, implications, and suggestions. Educ Rev (Birm). 72(1), pp. 41-67, 2020. DOI: https://doi.org/10.1080/00131911.2018.1483892
[27] Kilkenny, M.F., and Robinson, K.M., Data quality: “Garbage in – garbage out.” Health Information Management Journal. 47(3), pp. 103-105, 2018. DOI: https://doi.org/10.1177/1833358318774357
[28] Burgess, J., Buxton, N., Dyck, D., and Oosterveld, M., Thurston M., CIM Estimation best practice committee & mineral reserves best practices guidelines. Guidelines Specific to Particular Commodities Rock Hosted Diamonds. CIM Estimation Best Practice Committee. Published online, 2008.
[29] Abu-Mostafa, Y.S., Magdon-Ismail, M., and Lin, H.-T., Learning from data, AMLBook.com., vol. 1. AMLBook.com, 2012.
Cómo citar
IEEE
ACM
ACS
APA
ABNT
Chicago
Harvard
MLA
Turabian
Vancouver
Descargar cita
Licencia
Derechos de autor 2025 DYNA

Esta obra está bajo una licencia internacional Creative Commons Atribución-NoComercial-SinDerivadas 4.0.
El autor o autores de un artículo aceptado para publicación en cualquiera de las revistas editadas por la facultad de Minas cederán la totalidad de los derechos patrimoniales a la Universidad Nacional de Colombia de manera gratuita, dentro de los cuáles se incluyen: el derecho a editar, publicar, reproducir y distribuir tanto en medios impresos como digitales, además de incluir en artículo en índices internacionales y/o bases de datos, de igual manera, se faculta a la editorial para utilizar las imágenes, tablas y/o cualquier material gráfico presentado en el artículo para el diseño de carátulas o posters de la misma revista.