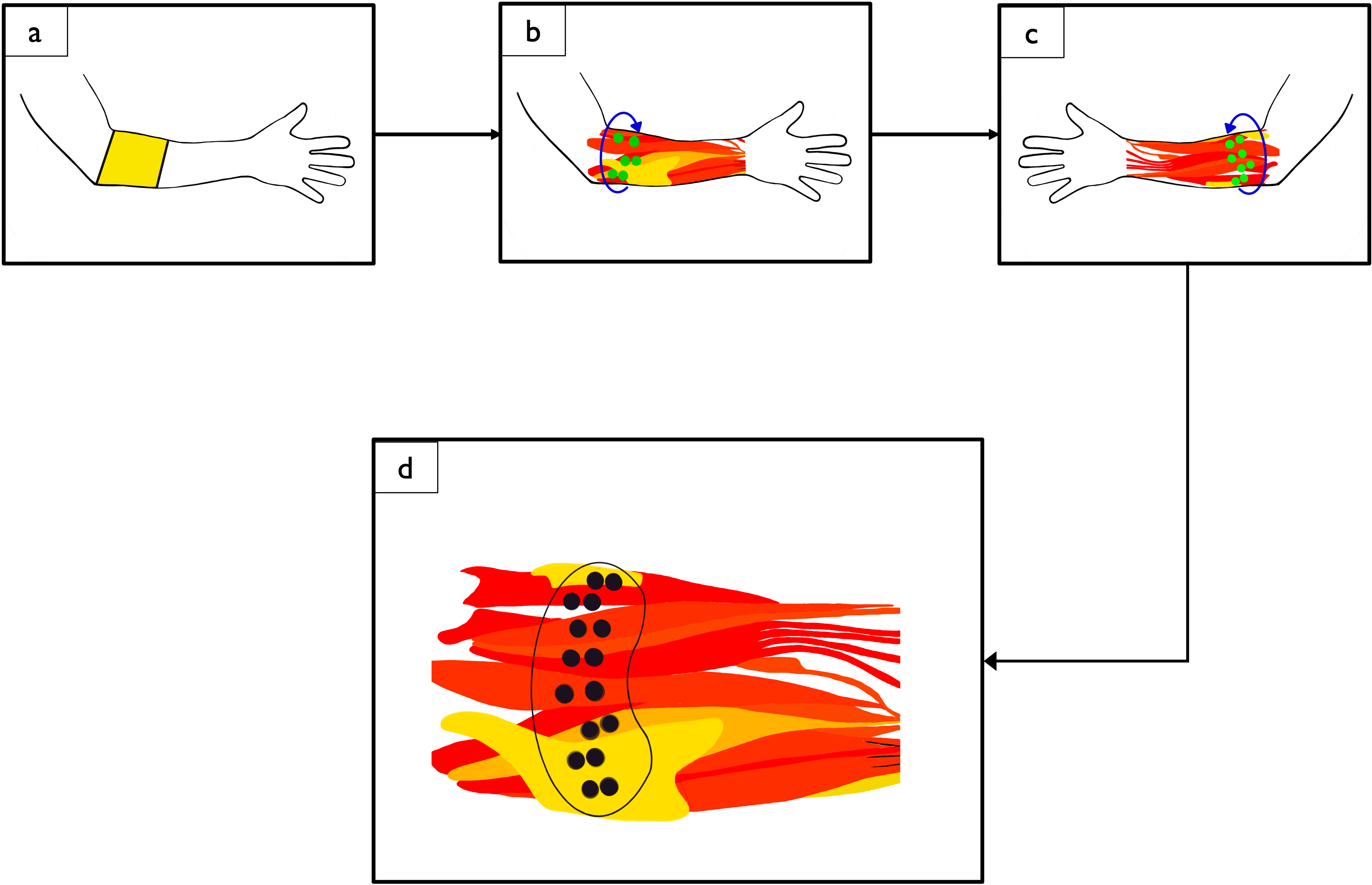
Published
Development and Validation of a Dry Electrode Array for sEMG Recording and Hand Movement Recognition
Desarrollo y validación de un arreglo de electrodos secos para la adquisición de señales sEMG y el reconocimiento de los movimientos de la mano
DOI:
https://doi.org/10.15446/ing.investig.106558Keywords:
dry electrodes, Bland-Altman, anatomical positioning array, hand movement classification (en)electrodos secos, Bland-Altman, arreglo de posicionamiento anatómico, clasificación de movimientos de la mano (es)
Downloads
Surface electromyography (sEMG) signals are an indirect measurement of muscle activity, and their applications range from biomechanics to control and rehabilitation. Hand movement recognition is a very difficult endeavor due to forearm anatomy. Hence, a multichannel approach for signal acquisition and processing is required. Conventional electrodes can limit the ease-of-use and repeatability of multi-channel sEMG recordings. New techniques have been proposed in this regard, with dry electrodes being one of them. Dry electrode technology has enabled the design of better donning and doffing procedures for multichannel sEMG recording, particularly for rehabilitation and prosthetic applications. However, there is a debate about the quality of the signals recorded with them and their usefulness for the recognition of multiple hand movements. To mitigate these quality issues, this work proposes an array of reusable stainless steel dry electrodes for multichannel sEMG recording with a design that facilitates its positioning on the forearm. The dry electrodes were characterized through electrical impedance measures and a Bland-Altman test. They were found to have similar characteristics to standard, disposable sEMG pre-gelled electrodes. For placement repeatability and application feasibility, an anatomy-based electrode positioning protocol was implemented with 17 healthy subjects and six hand movements. To evaluate the application feasibility of the electrode array, a feed-forward artificial neural network was trained to classify signals from the six movements, with a 97,86±0,58% accuracy. The amplitude of the sEMG signals for two antagonist movements was compared, finding a 24,81% variation. The dry electrode array showed feasibility in acquiring and classifying sEMG signals of hand movements with high accuracy.
Las señales de electromiografía de superficie (sEMG) son una medida indirecta de la actividad muscular, y sus aplicaciones van desde biomecánica hasta control y rehabilitación. La identificación de movimientos de la mano es una tarea muy complicada debido a la anatomía del antebrazo, por lo que se requiere un enfoque multicanal para adquisición y procesamiento de señales. Los electrodos convencionales pueden limitar la facilidad de uso y la repetibilidad de los registros sEMG multicanal. Se han propuesto nuevas técnicas para ello, entre ellas los electrodos secos. La tecnología de electrodos secos ha permitido el diseño de mejores procedimientos de colocación y remoción para registro sEMG multicanal, particularmente en aplicaciones de rehabilitación y prótesis. Sin embargo, existe un debate sobre la calidad de las señales registradas con ellos y su utilidad para el reconocimiento de múltiples movimientos de la mano. Para mitigar estos problemas de calidad, se propone un arreglo de electrodos secos reutilizables de acero inoxidable para registro sEMG multicanal con un diseño que facilita su posicionamiento en el antebrazo. Estos electrodos se caracterizaron mediante mediciones de impedancia eléctrica y una prueba Bland-Altman. Se encontró que tienen características similares a los electrodos pregelados desechables estándar para sEMG. Para la repetibilidad de la colocación y su viabilidad de aplicación, se implementó un protocolo de colocación de electrodos basado en la anatomía con 17 sujetos sanos y seis movimientos de la mano. Finalmente se entrenó una red neuronal artificial prealimentada para clasificar señales de los seis movimientos, con una precisión del 97,86±0,58 %. Se comparó la amplitud de las señales sEMG para dos movimientos antagonistas, encontrando una variación del 24,81 %. El arreglo de electrodos secos mostró viabilidad para adquirir y clasificar registros sEMG de los movimientos de la mano con gran precisión.
References
Abduo, M., and Galster, M. (2015). Myo gesture control armband for medical applications [Bachelor thesis, University of Canterbury]. http://hdl.handle.net/10092/14449
Al-Ayyad, M., Owida, H. A., De Fazio, R., Al-Naami, B., and Visconti, P. (2023). Electromyography monitoring systems in rehabilitation: A review of clinical applications, wearable devices and signal acquisition methodologies, Electronics (Switzerland), 12(7), 1520. https://doi.org/10.3390/electronics12071520 DOI: https://doi.org/10.3390/electronics12071520
Albulbul, A. (2016). Evaluating major electrode types for idle biological signal measurements for modern medical technology. Bioengineering, 3(3), 20. https://doi.org/10.3390/bioengineering3030020 DOI: https://doi.org/10.3390/bioengineering3030020
Barbero, M., Merletti, R., and Rainoldi, A. (2012a). Atlas of muscle innervation zones. Springer. https://doi.org/10.1007/978-88-470-2463-2 DOI: https://doi.org/10.1007/978-88-470-2463-2
Barbero, M., Merletti, R., and Rainoldi, A. (2012b). EMG imaging: Geometry and anatomy of the electrode-muscle system. In Springer (Eds.), Atlas of Muscle Innervation Zones (pp. 39-47). Springer. https://doi.org/10.1007/978-88-470-2463-2_4 DOI: https://doi.org/10.1007/978-88-470-2463-2_4
Besomi, M., Hodges, P. W., van Dieën, J., Carson, R. G., Clancy, E. A., Disselhorst-Klug, C., Holobar, A., Hug, F., Kiernan, M. C., Lowery, M., McGill, K., Merletti, R., Perreault, E., Søgaard, K., Tucker, K., Besier, T., Enoka, R., Falla, D., Farina, D., … Wrigley, T. (2019). Consensus for experimental design in electromyography (CEDE) project: Electrode selection matrix. Journal of Electromyography and Kinesiology, 48, 128-144. https://doi.org/10.1016/j.jelekin.2019.07.008 DOI: https://doi.org/10.1016/j.jelekin.2019.07.008
Biga, L. M., Dawson, S., Harwell, A., Hopkins, R., Kaufmann, J., LeMaster, M., Matern, P., Morrison-Graham, K., Quick, D., and Runyeon, J. (n.d.). Explain the organization of muscle fascicles and their role in generating force. https://open.oregonstate.education/aandp/chapter/11-2-explain-the-organization-of-muscle-fascicles-and-their-role-in-generating-force/#:~:text=When%20a%20group%20of%20muscle,the%20muscle's%20range%20of%20motion
Bland, J. M., and Altman, D. G. (1986). Statistical methods for assessing agreement between two methods of clinical measurement. Lancet, 1(8476), 307-310. DOI: https://doi.org/10.1016/S0140-6736(86)90837-8
Chen, L., Fu, J., Wu, Y., Li, H., and Zheng, B. (2020). Hand gesture recognition using compact CNN via surface electromyography signals. Sensors, 20(3), 672. https://doi.org/10.3390/s20030672 DOI: https://doi.org/10.3390/s20030672
Chi, Y. M., Jung, T.-P., and Cauwenberghs, G. (2010). Dry-contact and noncontact biopotential electrodes: Methodological review. IEEE Reviews in Biomedical Engineering, 3, 106-119. https://doi.org/10.1109/RBME.2010.2084078 DOI: https://doi.org/10.1109/RBME.2010.2084078
Dai, C., Zhu, Z., Martinez-Luna, C., Hunt, T. R., Farrell, T. R., and Clancy, E. A. (2019). Two degrees of freedom, dynamic, hand-wrist EMG-force using a minimum number of electrodes. Journal of Electromyography and Kinesiology, 47, 10-18. https://doi.org/10.1016/j.jelekin.2019.04.003 DOI: https://doi.org/10.1016/j.jelekin.2019.04.003
Gan, Y., Vauche, R., Pons, J. F., and Rahajandraibe, W. (2019). Dry electrode materials for electrocardiographic monitoring [Conference presentation]. 2018 25th IEEE International Conference on Electronics Circuits and Systems, Bourdeaux, France. https://doi.org/10.1109/ICECS.2018.8617992 DOI: https://doi.org/10.1109/ICECS.2018.8617992
Garcia, G. A., Zaccone, F., Ruff, R., Micera, S., Hoffmann, K.-P., and Dario, P. (2007). Characterization of a new type of dry electrodes for long-term recordings of surface-electromyogram [Conference presentation]. 2007 IEEE 10th International Conference on Rehabilitation Robotics, Noordwijk, Netherlands. https://doi.org/10.1109/ICORR.2007.4428523 DOI: https://doi.org/10.1109/ICORR.2007.4428523
Ghoshdastider, U., Lange, C., Viga, R., and Grabmaier, A. (2012). A modular and wireless exg signal acquisition system with a dense array of dry electrodes [Conference presentation]. IEEE Sensors 2012, Taipei, Taiwan. https://doi.org/10.1109/ICSENS.2012.6411473 DOI: https://doi.org/10.1109/ICSENS.2012.6411473
Guo, L., Sandsjö, L., Ortiz-Catalan, M., and Skrifvars, M. (2020). Systematic review of textile-based electrodes for long-term and continuous surface electromyography recording. Textile Research Journal, 90(2), 227-244. https://doi.org/10.1177/0040517519858768 DOI: https://doi.org/10.1177/0040517519858768
Guzmán, R. A., Silvestre, R. A., and Arriagada, D. A. (2011). Biceps brachii muscle innervation zone location in healthy subjects using high-density surface electromyography. International Journal of Morphology, 29(2), 347-352. https://doi.org/10.4067/s0717-95022011000200007 DOI: https://doi.org/10.4067/S0717-95022011000200007
Hall, J. E. (2011). Guyton & Hall: tratado de fisiología medica. Elsevier.
Hermens, H. J., Freriks, B., Disselhorst-Klug, C., and Rau, G. (2000). Development of recommendations for SEMG sensors and sensor placement procedures. Journal of Electromyography and Kinesiology: Official Journal of the International Society of Electrophysiological Kinesiology, 10(5), 361-374. https://doi.org/10.1016/s1050-6411(00)00027-4 DOI: https://doi.org/10.1016/S1050-6411(00)00027-4
Kang, S., Kim, H., Park, C., Sim, Y., Lee, S., and Jung, Y. (2023). sEMG-based hand gesture recognition using binarized neural network. Sensors, 23(3), 1436. https://doi.org/10.3390/s23031436 DOI: https://doi.org/10.3390/s23031436
Kapelner, T., Jiang, N., Holobar, A., Vujaklija, I., Roche, A. D., Farina, D., and Aszmann, O. C. (2016). Motor unit characteristics after targeted muscle reinnervation. PLoS ONE, 11(2), 0149772. https://doi.org/10.1371/journal.pone.0149772 DOI: https://doi.org/10.1371/journal.pone.0149772
Kusche, R., Kaufmann, S., and Ryschka, M. (2019). Dry electrodes for bioimpedance measurements - Design, characterization and comparison. Biomedical Physics and Engineering Express, 5(1), 015001. https://doi.org/10.1088/2057-1976/aaea59 DOI: https://doi.org/10.1088/2057-1976/aaea59
Lee, H., Lee, S., Kim, J., Jung, H., Yoon, K. J., Gandla, S., Park, H., and Kim, S. (2023). Stretchable array electromyography sensor with graph neural network for static and dynamic gestures recognition system. Npj Flexible Electronics, 7(1), 20. https://doi.org/10.1038/s41528-023-00246-3 DOI: https://doi.org/10.1038/s41528-023-00246-3
Liu, J. (2015). Adaptive myoelectric pattern recognition toward improved multifunctional prosthesis control. Medical Engineering and Physics, 37(4), 424-430. https://doi.org/10.1016/j.medengphy.2015.02.005 DOI: https://doi.org/10.1016/j.medengphy.2015.02.005
Losier, Y., Clawson, W., Scheme, E., Englehart, K., Kyberd, P., and Hudgins, B. (2011). An overview of the UNB hand system [Conference presentation]. MyoElectric Controls/Powered Prosthetics Symposium Fredericton, NB, Canada.
Martin, A. I., Toledo, C., Mercado, J. A., Vera, A., Leija, L., and Gutierrez, J. (2018). Evaluation of dry electrodes for sEMG recording [Conference presentation]. 2018 Global Medical Engineering Physics Exchanges/Pan American Health Care Exchanges (GMEPE/PAHCE), Porto, Portugal. https://doi.org/10.1109/GMEPE-PAHCE.2018.8400758 DOI: https://doi.org/10.1109/GMEPE-PAHCE.2018.8400758
Merletti, R., and Farina, D. (Eds.) (2016). Surface electromyography physiology, engineering, and applications. John Wiley & Sons. DOI: https://doi.org/10.1002/9781119082934
Meziane, N., Webster, J. G., Attari, M., and Nimunkar, A. J. (2013). Dry electrodes for electrocardiography. Physiological Measurement, 34(9), R47-R69. https://doi.org/10.1088/0967-3334/34/9/R47 DOI: https://doi.org/10.1088/0967-3334/34/9/R47
Mitchell, B., & Whited, L. (2019). Anatomy, shoulder and upper limb, forearm muscles. https://www.ncbi.nlm.nih.gov/books/NBK536975/
Moin, A., Zhou, A., Rahimi, A., Benatti, S., Menon, A., Tamakloe, S., Ting, J., Yamamoto, N., Khan, Y., Burghardt, F., Benini, L., Arias, A. C., and Rabaey, J. M. (2018). An EMG gesture recognition system with flexible high-density sensors and brain-inspired high-dimensional classifier [Conference presentation]. IEEE International Symposium on Circuits and Systems, Florence, Italy. https://doi.org/10.1109/ISCAS.2018.8351613 DOI: https://doi.org/10.1109/ISCAS.2018.8351613
Morais, G. D., Neves, L. C., Masiero, A. A., and Castro, M. C. F. (2016). Application of Myo Armband System to control a robot interface [Conference presentation]. BIOSIGNALS 2016 - 9th International Conference on Bio-Inspired Systems and Signal Processing, Rome, Italy. https://doi.org/10.5220/0005706302270231 DOI: https://doi.org/10.5220/0005706302270231
Niu, X., Gao, X., Liu, Y., and Liu, H. (2021). Surface bioelectric dry Electrodes: A review. Measurement: Journal of the International Measurement Confederation, 183, 109774. https://doi.org/10.1016/j.measurement.2021.109774 DOI: https://doi.org/10.1016/j.measurement.2021.109774
Palumbo, A., Vizza, P., Calabrese, B., and Ielpo, N. (2021). Biopotential signal monitoring systems in rehabilitation: A review. Sensors, 21(21), 7172. https://doi.org/10.3390/s21217172 DOI: https://doi.org/10.3390/s21217172
Peng, Y., He, J., Yao, B., Li, S., Zhou, P., and Zhang, Y. (2016). Motor unit number estimation based on high-density surface electromyography decomposition. Clinical Neurophysiology, 127(9), 3059-3065. https://doi.org/10.1016/j.clinph.2016.06.014 DOI: https://doi.org/10.1016/j.clinph.2016.06.014
Phinyomark, A., Quaine, F., Charbonnier, S., Serviere, C., Tarpin-Bernard, F., and Laurillau, Y. (2013). EMG feature evaluation for improving myoelectric pattern recognition robustness. Expert Systems with Applications, 40(12), 4832-4840. https://doi.org/10.1016/j.eswa.2013.02.023 DOI: https://doi.org/10.1016/j.eswa.2013.02.023
Phinyomark, A., Quaine, F., Charbonnier, S., Serviere, C., Tarpin-Bernard, F., and Laurillau, Y. (2014). Feature extraction of the first difference of EMG time series for EMG pattern recognition. Computer Methods and Programs in Biomedicine, 117(2), 247-256. https://doi.org/10.1016/j.cmpb.2014.06.013 DOI: https://doi.org/10.1016/j.cmpb.2014.06.013
Pizzolato, S., Tagliapietra, L., Cognolato, M., Reggiani, M., Müller, H., and Atzori, M. (2017). Comparison of six electromyography acquisition setups on hand movement classification tasks. PLoS ONE, 12(10), 0186132. https://doi.org/10.1371/journal.pone.0186132 DOI: https://doi.org/10.1371/journal.pone.0186132
Puurtinen, M. M., Komulainen, S. M., Kauppinen, P. K., Malmivuo, J. A. V., and Hyttinen, J. A. K. (2006). Measurement of noise and impedance of dry and wet textile electrodes, and textile electrodes with hydrogel [Conference presentation]. 2006 International Conference of the IEEE Engineering in Medicine and Biology Society, New York, NY, USA. https://doi.org/10.1109/IEMBS.2006.260155 DOI: https://doi.org/10.1109/IEMBS.2006.260155
Reategui, J., and Callupe, R. (2017). Surface EMG multichannel array using active dry sensors for forearm signal extraction [Conference presentation]. 2017 IEEE 24th International Congress on Electronics, Electrical Engineering and Computing, Cusco, Peru. https://doi.org/10.1109/INTERCON.2017.8079699 DOI: https://doi.org/10.1109/INTERCON.2017.8079699
“Recommendations for the practice of clinical neurophysiology: Guidelines of the International Federation of Clinical Neurophysiology” (1999). Electroencephalography and Clinical Neurophysiology, 52(supp.), 1-304.
Rodrigues, M. S., Fiedler, P., Küchler, N., Domingues, R. P., Lopes, C., Borges, J., Haueisen, J., and Vaz, F. (2020). Dry electrodes for surface electromyography based on architectured titanium thin films. Materials, 13(9), 2135. https://doi.org/10.3390/ma13092135 DOI: https://doi.org/10.3390/ma13092135
Rojas-Martínez, M., Mañanas, M. A., Alonso, J. F., and Merletti, R. (2013). Identification of isometric contractions based on High Density EMG maps. Journal of Electromyography and Kinesiology, 23(1), 33-42. https://doi.org/10.1016/j.jelekin.2012.06.009 DOI: https://doi.org/10.1016/j.jelekin.2012.06.009
Rui Ma, Dae-Hyeong Kim, McCormick, M., Coleman, T., and Rogers, J. (2010). A stretchable electrode array for non-invasive, skin-mounted measurement of electrocardiography (ECG), electromyography (EMG) and electroencephalography (EEG) [Conference presentation]. 2010 Annual International Conference of the IEEE Engineering in Medicine and Biology, Buenos Aires, Argentina. https://doi.org/10.1109/IEMBS.2010.5627315 DOI: https://doi.org/10.1109/IEMBS.2010.5627315
Ruvalcaba, A., Altamirano, A., Toledo, C., Munoz, R., Vera, A., and Leija, L. (2016). Design and measurement of the standards of a miniaturized sEMG acquisition system with dry electrodes integrated [Conference presentation]. 2015 International Conference on Mechatronics, Electronics, and Automotive Engineering, Cuernavaca, Mexico. https://doi.org/10.1109/ICMEAE.2015.34 DOI: https://doi.org/10.1109/ICMEAE.2015.34
Ruvalcaba, A., Muñoz, R., Vera, A., and Leija, L. (2017a). Design and test of a dry electrode array implemented on wearable sEMG acquisition sleeve for long term monitoring [Conference presentation]. Pan American Health Care Exchanges, PAHCE, 2017-March. https://doi.org/10.1109/GMEPE-PAHCE.2017.7972111 DOI: https://doi.org/10.1109/GMEPE-PAHCE.2017.7972111
Srinivasa, M. G., and Pandian, P. S. (2017). Dry electrodes for bio-potential measurement in wearable systems [Conference presentation]. 2017 2nd IEEE International Conference on Recent Trends in Electronics, Information & Communication Technology (RTEICT), Bangalore, India. https://doi.org/10.1109/RTEICT.2017.8256600 DOI: https://doi.org/10.1109/RTEICT.2017.8256600
Tepe, C., and Demir, M. C. (2022). Real-time classification of EMG Myo Armband data using support vector machine. IRBM, 43(4), 300-308. https://doi.org/10.1016/j.irbm.2022.06.001 DOI: https://doi.org/10.1016/j.irbm.2022.06.001
Toledo, C., Flores, E., Mercado, J., Castellanos, P., and Gutiérrez, J. (2018, September 26). Multiclass sEMG signal processing and classification for upper-limb FES-NP control [Conference presentation]. Annual Conference of the German Society for Biomedical Engineering, Aachen, Germany.
Toledo-Peral, C. L., Gutiérrez-Martínez, J., Mercado-Gutiérrez, J. A., Martín-Vignon-Whaley, A. I., Vera-Hernández, A., and Leija-Salas, L. (2018). sEMG Signal Acquisition Strategy towards Hand FES Control. Journal of Healthcare Engineering, 2018, 2350834. https://doi.org/10.1155/2018/2350834 DOI: https://doi.org/10.1155/2018/2350834
Toledo-Peral, C. L., Vega-Martínez, G., Mercado-Gutiérrez, J. A., Rodríguez-Reyes, G., Vera-Hernández, A., Leija-Salas, L., and Gutiérrez-Martínez, J. (2022). Virtual/augmented reality for rehabilitation applications using electromyography as control/biofeedback: Systematic literature review. Electronics, 11(14), 2271. https://doi.org/10.3390/electronics11142271 DOI: https://doi.org/10.3390/electronics11142271
Topalović, I., Graovac, S., and Popović, D. B. (2019). EMG map image processing for recognition of fingers movement. Journal of Electromyography and Kinesiology, 49, 102364. https://doi.org/10.1016/j.jelekin.2019.102364 DOI: https://doi.org/10.1016/j.jelekin.2019.102364
Urbanek, H., and van der Smagt, P. (2016). IEMG: Imaging electromyography. Journal of Electromyography and Kinesiology, 27, 1-9. https://doi.org/10.1016/j.jelekin.2016.01.001 DOI: https://doi.org/10.1016/j.jelekin.2016.01.001
Visconti, P., Gaetani, F., Zappatore, G. A., and Primiceri, P. (2018). Technical features and functionalities of Myo armband: An overview on related literature and advanced applications of myoelectric armbands mainly focused on arm prostheses. International Journal on Smart Sensing and Intelligent Systems, 11(1), 1-25. https://doi.org/10.21307/ijssis-2018-005 DOI: https://doi.org/10.21307/ijssis-2018-005
Vojtech, L., Bortel, R., Neruda, M., and Kozak, M. (2013). Wearable textile electrodes for ECG measurement. Advances in Electrical and Electronic Engineering, 11(5), 410-414. https://doi.org/10.15598/aeee.v11i5.889 DOI: https://doi.org/10.15598/aeee.v11i5.889
Xie, L. Yang, G., Xu, L., Seoane, F., Chen, Q., and Zheng, L. (2013). Characterization of dry biopotential electrodes [Conference presentation]. 2013 35th Annual International Conference of the IEEE Engineering in Medicine and Biology Society (EMBC), Osaka, Japan. https://doi.org/10.1109/EMBC.2013.6609791 DOI: https://doi.org/10.1109/EMBC.2013.6609791
Yakob, M. Y. bin, Baharuddin, M. Z. bin, Khairudin, A. R. M., and Karim, M. H. B. A. (2021). Telecontrol of prosthetic robot hand using Myo Armband [Conference presentation]. 2021 IEEE International Conference on Automatic Control and Intelligent Systems, I2CACIS 2021, Shah Alam, Malaysia. https://doi.org/10.1109/I2CACIS52118.2021.9495919 DOI: https://doi.org/10.1109/I2CACIS52118.2021.9495919
Young, A. J., Hargrove, L. J., and Kuiken, T. A. (2011). The Effects of Electrode Size and Orientation on the Sensitivity of Myoelectric Pattern Recognition Systems to Electrode Shift. IEEE Transactions on Biomedical Engineering, 58(9), 2537-2544. https://doi.org/10.1109/TBME.2011.2159216 DOI: https://doi.org/10.1109/TBME.2011.2159216
How to Cite
APA
ACM
ACS
ABNT
Chicago
Harvard
IEEE
MLA
Turabian
Vancouver
Download Citation
CrossRef Cited-by
Dimensions
PlumX
Article abstract page views
Downloads
License
Copyright (c) 2024 Cinthya Lourdes Toledo Peral, Ana Isabel Martín-Vignon-Whaley, Jorge Airy Mercado-Gutierrez, Arturo Vera-Hernandez, Lorenzo Leija-Salas, Josefina Gutierrez-Martinez

This work is licensed under a Creative Commons Attribution 4.0 International License.
The authors or holders of the copyright for each article hereby confer exclusive, limited and free authorization on the Universidad Nacional de Colombia's journal Ingeniería e Investigación concerning the aforementioned article which, once it has been evaluated and approved, will be submitted for publication, in line with the following items:
1. The version which has been corrected according to the evaluators' suggestions will be remitted and it will be made clear whether the aforementioned article is an unedited document regarding which the rights to be authorized are held and total responsibility will be assumed by the authors for the content of the work being submitted to Ingeniería e Investigación, the Universidad Nacional de Colombia and third-parties;
2. The authorization conferred on the journal will come into force from the date on which it is included in the respective volume and issue of Ingeniería e Investigación in the Open Journal Systems and on the journal's main page (https://revistas.unal.edu.co/index.php/ingeinv), as well as in different databases and indices in which the publication is indexed;
3. The authors authorize the Universidad Nacional de Colombia's journal Ingeniería e Investigación to publish the document in whatever required format (printed, digital, electronic or whatsoever known or yet to be discovered form) and authorize Ingeniería e Investigación to include the work in any indices and/or search engines deemed necessary for promoting its diffusion;
4. The authors accept that such authorization is given free of charge and they, therefore, waive any right to receive remuneration from the publication, distribution, public communication and any use whatsoever referred to in the terms of this authorization.